面向我国化学物质环境管理的计算毒理学模型评估思路及建设路径
Evaluation Thoughts of Computational Toxicological Models and Constructional Path for Environmental Management of Chemicals in China
-
摘要: 计算毒理学模型正逐渐成为我国化学物质环境管理的重要工具之一。为了推动建立面向我国化学物质环境管理的计算毒理学模型评估方法体系,掌握模型评估核心技术,本文分析了我国发展和评估计算毒理学模型的必要性,总结了我国计算毒理学模型评估的管理需求,系统梳理了美国、欧盟、国际经济合作与发展组织(OECD)的模型评估方法。研究发现,发达国家普遍建立了一套相对完善的计算毒理学模型评估技术,构建了包含模型开发、评估、验证和应用的模型生命周期评估体系。当前,我国尚未建立计算毒理学模型评估技术方法,借鉴发达国家的经验做法,构建具有我国国情特征的计算毒理学模型评估体系,指导我国计算毒理学模型征集与评估工作,具有重要意义。Abstract: Computational toxicological models are gradually becoming one of the most important tools for chemical environmental management in China. In order to promote the establishment of the evaluation method system of computational toxicology models for chemical environmental management in China and master the core technology of model evaluation, this paper analyzed the necessity of developing and evaluating computational toxicology models, summarized the management requirements of computational toxicology model evaluation in China, and systematically reviewed the model evaluation methods of the United States, the European Union and the Organization for Economic Co-operation and Development (OECD). It was found that in developed countries, a set of relatively perfect computational toxicology model assessment technology had been established, and a model life cycle assessment system including model development, assessment, validation and application had been constructed. At present, computational toxicology model evaluation techniques of China has not been established. It is of great significance to build a computational toxicology model evaluation system based on the experience of developed countries, and to guide the collection and evaluation of computational toxicology models in China.
-
Key words:
- computational toxicology /
- model /
- evaluation thoughts /
- constructional path
-
-
Sizochenko N, Syzochenko M, Fjodorova N, et al. Evaluating genotoxicity of metal oxide nanoparticles:Application of advanced supervised and unsupervised machine learning techniques[J]. Ecotoxicology and Environmental Safety, 2019, 185:109733 Joint Research Centre. JRC QSAR model database-Guideline for authors and editors[R]. Brussel:Joint Research Centre, 2017 Organization for Economic Co-operation and Development(OECD). The report from the expert group on (quantitative) structure-activity relationships on the principles for the validation of (Q)SARs[R]. Paris:OECD, 2004 Minerali E, Foil D H, Zorn K M, et al. Evaluation of assay central machine learning models for rat acute oral toxicity prediction[J]. ACS Sustainable Chemistry & Engineering, 2020, 8(42):16020-16027 中华人民共和国生态环境部. 新化学物质环境管理登记办法[S]. 北京:中华人民共和国生态环境部, 2020 中华人民共和国生态环境部. 中国现有化学物质名录[S]. 北京:中华人民共和国生态环境部, 2020 Bouhedjar K, Benfenati E, Nacereddine A K. Modelling quantitative structure activity-activity relationships (QSAARs):Auto-pass-pass, a new approach to fill data gaps in environmental risk assessment under the REACH regulation[J]. SAR and QSAR in Environmental Research, 2020, 31(10):785-801 中华人民共和国生态环境部. 新化学物质环境管理登记指南[S]. 北京:中华人民共和国生态环境部, 2020 Karcher W, Hansen B G, Leeuwen C V, et al. Predictions for existing chemicals-A multilateral QSAR project[J]. SAR and QSAR in Environmental Research, 1995, 3(3):217-221 United States Environmental Protection Agency (US EPA). Frank R. Lautenberg Chemical Safety for the 21st Century Act[S]. Washington DC:US EPA, 2016 United States Environmental Protection Agency (US EPA). Guidance on the development, evaluation, and application of environmental models[S]. Washington DC:US EPA, 2009 United States Environmental Protection Agency (US EPA). Best modeling practices-Model development[R]. Washington DC:US EPA, 2009 United States Environmental Protection Agency (US EPA). Best modeling practices-Model evaluation[R]. Washington DC:US EPA, 2009 United States Environmental Protection Agency (US EPA). Best modeling practices-Model application[R]. Washington DC:US EPA, 2009 United States Environmental Protection Agency (US EPA). Best modeling practices-The model life-cycle[R]. Washington DC:US EPA, 2009 Jaworska J S, Comber M, Auer C, et al. Summary of a workshop on regulatory acceptance of (Q)SARs for human health and environmental endpoints[J]. Environmental Health Perspectives, 2003, 111(10):1358-1360 Organization for Economic Co-operation and Development (OECD). Guidance document on the validation of (quantitative) structure-activity relationship models[R]. Paris:OECD, 2014 Zind H, Mondamert L, Remaury Q B, et al. Occurrence of carbamazepine, diclofenac, and their related metabolites and transformation products in a French aquatic environment and preliminary risk assessment[J]. Water Research, 2021, 196:117052 Joint Research Centre. JRC QSAR model database-Guideline and protocol template for reviewers[R]. Brussel:Joint Research Centre, 2017 于洋, 左平春, 张楠, 等. 计算毒理学在农药风险评估中的应用[J]. 农药科学与管理, 2017, 38(4):24-30 Yu Y, Zuo P C, Zhang N, et al. Application of computational toxicology in risk assessment of pesticide[J]. Pesticide Science and Administration, 2017, 38(4):24-30(in Chinese)
于洋, 郑永权, 张楠, 等. 蔬菜常用农药在地下水中残留风险评估[J]. 生态毒理学报, 2017, 12(4):183-192 Yu Y, Zheng Y Q, Zhang N, et al. Groundwater risk assessment of pesticides commonly used on vegetables[J]. Asian Journal of Ecotoxicology, 2017, 12(4):183-192(in Chinese)
左平春, 于洋, 张楠, 等. 农药环境风险评估中常用的计算毒理学模型软件[J]. 生态毒理学报, 2017, 12(4):98-109 Zuo P C, Yu Y, Zhang N, et al. Commonly-used model softwares of computational toxicology in pesticide environmental risk assessment[J]. Asian Journal of Ecotoxicology, 2017, 12(4):98-109(in Chinese)
-
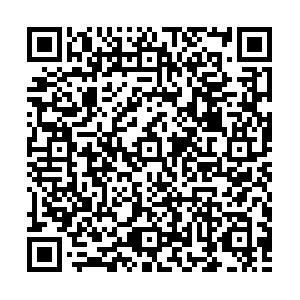
计量
- 文章访问数: 5130
- HTML全文浏览数: 5130
- PDF下载数: 147
- 施引文献: 0