大数据挖掘和机器学习在毒理学中的应用
Application of Data Mining and Machine Learning in Toxicology
-
摘要: 随着高通量筛选技术的快速发展,化学品的毒性相关信息与日俱增。现今快速发展的数据挖掘技术和机器学习等计算机方法为化学品的毒性预测和风险防控提供了新途径。有害结局路径(adverse outcome pathway,AOP)将化合物的结构、分子启动事件和生物的有害结局建立关联,为污染物的毒性测试、预测和评估提供了新的模式,最终实现风险评估并应用于管理决策。定量结构-活性关系(QSAR)建模、分子模拟以及多组学技术在AOP的各个方面发挥了重要作用。基于此,本综述主要介绍数据挖掘与机器学习在毒理学中的应用方法,涉及QSAR建模、分子模拟及组学等方面,并结合实例分析系统阐述了当前研究的重点与方向,以更好地适应当前大数据时代的研究背景。Abstract: With the rapid development of high-throughput screening technologies, information on the toxicity of chemicals is growing day by day. The rapid development of computerized methods, such as data mining and machine learning, has provided a new approach to the toxicity prediction and risk control of chemicals. It is very important to establish the framework of ecological risk assessment by integrating a series of effective tools. Among these tools, adverse outcome pathway (AOP) can connect the structure of compounds, molecular initiation events, and adverse effects of organisms, thus can be used for risk assessment and management decisions. Quantitative structure-activity relationship (QSAR) modeling, molecular simulation and multi-omics techniques play important roles in the function of AOP. This review mainly introduces the application methods of data mining and machine learning in toxicology, including QSAR modeling, molecular simulation and omics. The current research focus and direction of computational toxicology were also reviewed with the aim of the better understanding of the big data era.
-
Key words:
- data mining /
- machine learning /
- structure-activity relationship /
- AOP /
- computational toxicology
-
-
Stokes W. The interagency coordinating committee on the validation of alternative methods (ICCVAM):Recent progress in the evaluation of alternative toxicity testing methods[R]. Bethesda:NTP Interagency Center for the Evaluation of Alternative Toxicological Methods, 2017 郭家彬,彭双清.动物实验替代方法与21世纪毒性测试发展策略[J].中国比较医学杂志, 2011, 21(S1):157-161 , 156Guo J B, Peng S Q. Animal alternative methods and the development of strategy for toxicity testing in the Twenty-First Century[J]. Chinese Journal of Comparative Medicine, 2011, 21(S1):157-161, 156(in Chinese)
王中钰,陈景文,乔显亮,等.面向化学品风险评价的计算(预测)毒理学[J].中国科学:化学, 2016, 46(2):222-240 Wang Z Y, Chen J W, Qiao X L, et al. Computational toxicology:Oriented for chemicals risk assessment[J]. Scientia Sinica Chimica, 2016, 46(2):222-240(in Chinese)
Card M L, Gomez-Alvarez V, Lee W H, et al. History of EPI SuiteTM and future perspectives on chemical property estimation in US Toxic Substances Control Act new chemical risk assessments[J]. Environmental Science Processes&Impacts, 2017, 19(3):203-212 Dimitrov S D, Diderich R, Sobanski T, et al. QSAR toolbox:Workflow and major functionalities[J]. SAR and QSAR in Environmental Research, 2016, 27(3):203-219 Fatoyinbo T, Rincon R F, Sun G Q, et al. Ecosar:A P-band digital beamforming polarimetric interferometric SAR instrument to measure ecosystem structure and biomass[C]//Vancouver, BC, Canada:IEEE International Geoscience and Remote Sensing Symposium, 2011:1524-1527 Tice R R, Austin C P, Kavlock R J, et al. Improving the human hazard characterization of chemicals:A Tox21 update[J]. Environmental Health Perspectives, 2013, 121(7):756-765 Shukla S J, Huang R L, Austin C P, et al. The future of toxicity testing:A focus on in vitro methods using a quantitative high-throughput screening platform[J]. Drug Discovery Today, 2010, 15(23-24):997-1007 Sturla S J, Boobis A R, FitzGerald R E, et al. Systems toxicology:From basic research to risk assessment[J]. Chemical Research in Toxicology, 2014, 27(3):314-329 李杰,李柯佳,张臣,等.计算系统毒理学:形成、发展及应用[J].科学通报, 2015, 60(19):1751-1761 Li J, Li K J, Zhang C, et al. Computational systems toxicology:Emergence, development and application[J]. Chinese Science Bulletin, 2015, 60(19):1751-1761(in Chinese)
Ankley G T, Bennett R S, Erickson R J, et al. Adverse outcome pathways:A conceptual framework to support ecotoxicology research and risk assessment[J]. Environmental Toxicology and Chemistry, 2010, 29(3):730-741 Jagiello K, Halappanavar S, Rybińska-Fryca A, et al. Transcriptomics-based and AOP-informed structure-activity relationships to predict pulmonary pathology induced by multiwalled carbon nanotubes[J]. Small, 2021, 17(15):e2003465 Hu M Y, Palic D A. Micro-and nano-plastics activation of oxidative and inflammatory adverse outcome pathways[J]. Redox Biology, 2020, 37:101620 Rugard M, Coumoul X, Carvaillo J C, et al. Deciphering adverse outcome pathway network linked to bisphenol F using text mining and systems toxicology approaches[J]. Toxicological Sciences:An Official Journal of the Society of Toxicology, 2020, 173(1):32-40 Jordan M I, Mitchell T M. Machine learning:Trends, perspectives, and prospects[J]. Science, 2015, 349(6245):255-260 Lu S H, Zhou Q H, Ouyang Y X, et al. Accelerated discovery of stable lead-free hybrid organic-inorganic perovskites via machine learning[J]. Nature Communications, 2018, 9(1):3405 Caruana R, Niculescu-Mizil A. An empirical comparison of supervised learning algorithms[C]//Proceedings of the 23rd International Conference on Machine Learning. Pittsburgh:ACM Press, 2006 Sathya R, Abraham A. Comparison of supervised and unsupervised learning algorithms for pattern classification[J]. International Journal of Advanced Research in Artificial Intelligence, 2013, 2(2):34-38 Hu J Y, Niu H L, Carrasco J, et al. Voronoi-based multi-robot autonomous exploration in unknown environments via deep reinforcement learning[J]. IEEE Transactions on Vehicular Technology, 2020, 69(12):14413-14423 Qin R, Wang H, Yan A. Classification and QSAR models of leukotriene A4 hydrolase (LTA4H) inhibitors by machine learning methods[J]. SAR and QSAR in Environmental Research, 2021, 32(5):411-431 Ha M K, Trinh T X, Choi J S, et al. Toxicity classification of oxide nanomaterials:Effects of data gap filling and PChem score-based screening approaches[J]. Scientific Reports, 2018, 8(1):3141 Furxhi I, Murphy F, Poland C A, et al. Application of Bayesian networks in determining nanoparticle-induced cellular outcomes using transcriptomics[J]. Nanotoxicology, 2019, 13(6):827-848 Drgan V, Bajželj B. Application of supervised SOM algorithms in predicting the hepatotoxic potential of drugs[J]. International Journal of Molecular Sciences, 2021, 22(9):4443 Ge Z Q, Song Z H, Ding S X, et al. Data mining and analytics in the process industry:The role of machine learning[J]. IEEE Access, 2017, 5:20590-20616 United States Environmental Protection Agency (US EPA). Exploring ToxCast Data[EB/OL].[2021-09-15]. https://www.epa.gov/chemical-research/exploring-toxcast-data-downloadable-data United States Environmental Protection Agency (US EPA). ACToR Safer Chemicals Research[EB/OL].[2021-09-15]. https://19january2017snapshot.epa.gov/chemical-research/actor.html European Bioinformatics Institute. ChEMBL:A large-scale bioactivity database for drug discovery[EB/OL].[2021-09-15]. https://www.ebi.ac.uk/chembl/ Bioinfogate. OFF-X Website[EB/OL].[2021-09-15]. https://www.targetsafety.info/ National Library of Medicine. PubChem:A public information system for analyzing bioactivities of small molecules.[EB/OL].[2021-09-15]. https://pubchem.ncbi.nlm.nih.gov/ Wishart D S. DrugBank Online. Database for Drug and Drug Target Info.[EB/OL].[2021-09-15]. https://go.drugbank.com/ United States Environmental Protection Agency (US EPA). ECOTOX Knowledgebase[EB/OL].[2021-09-15]. https://cfpub.epa.gov/ecotox/ National Institute of Environmental Health Sciences. The Comparative Toxicogenomics Database[EB/OL].[2021-09-15]. https://ctdbase.org/ Cherkasov A, Muratov E N, Fourches D, et al. QSAR modeling:Where have You been?Where are you going to?[J]. Journal of Medicinal Chemistry, 2014, 57(12):4977-5010 Tang W H, Chen J W, Hong H X. Discriminant models on mitochondrial toxicity improved by consensus modeling and resolving imbalance in training[J]. Chemosphere, 2020, 253:126768 Capuzzi S J, Politi R, Isayev O, et al. QSAR modeling of Tox21 challenge stress response and nuclear receptor signaling toxicity assays[J]. Frontiers in Environmental Science, 2016, 4:3 Cao Q Q, Liu L, Yang H B, et al. in silico estimation of chemical aquatic toxicity on crustaceans using chemical category methods[J]. Environmental Science Processes&Impacts, 2018, 20(9):1234-1243 Vegosen L, Martin T M. An automated framework for compiling and integrating chemical hazard data[J]. Clean Technologies and Environmental Policy, 2020, 22(2):441-458 Yu F B, Wei C H, Deng P, et al. Deep exploration of random forest model boosts the interpretability of machine learning studies of complicated immune responses and lung burden of nanoparticles[J]. Science Advances, 2021, 7(22):eabf4130 Rabinowitz J R, Goldsmith M R, Little S B, et al. Computational molecular modeling for evaluating the toxicity of environmental chemicals:Prioritizing bioassay requirements[J]. Environmental Health Perspectives, 2008, 116(5):573-577 Walden D M, Bundey Y, Jagarapu A, et al. Molecular simulation and statistical learning methods toward predicting drug-polymer amorphous solid dispersion miscibility, stability, and formulation design[J]. Molecules, 2021, 26(1):E182 Mazurek A H, Szeleszczuk , Pisklak D M. A review on combination of ab initio molecular dynamics and NMR parameters calculations[J]. International Journal of Molecular Sciences, 2021, 22(9):4378 Li J, Cao H M, Feng H R, et al. Evaluation of the estrogenic/antiestrogenic activities of perfluoroalkyl substances and their interactions with the human estrogen receptor by combining in vitro assays and in silico modeling[J]. Environmental Science&Technology, 2020, 54(22):14514-14524 Xue Q, Liu X, Liu X C, et al. The effect of structural diversity on ligand specificity and resulting signaling differences of estrogen receptor Α[J]. Chemical Research in Toxicology, 2019, 32(6):1002-1013 Cao H M, Wang L, Liang Y, et al. Protonation state effects of estrogen receptor α on the recognition mechanisms by perfluorooctanoic acid and perfluorooctane sulfonate:A computational study[J]. Ecotoxicology and Environmental Safety, 2019, 171:647-656 de Araujo A S, Martínez L, de Paula Nicoluci R, et al. Structural modeling of high-affinity thyroid receptor-ligand complexes[J]. European Biophysics Journal, 2010, 39(11):1523-1536 Subramaniam S, Mehrotra M, Gupta D. Virtual high throughput screening (vHTS):A perspective[J]. Bioinformation, 2008, 3(1):14-17 Troger F, Delp J, Funke M, et al. Identification of mitochondrial toxicants by combined in silico and in vitro studies:A structure-based view on the adverse outcome pathway[J]. Computational Toxicology, 2020, 14:100123 Kanehisa M, Bork P. Bioinformatics in the post-sequence era[J]. Nature Genetics, 2003, 33(Suppl.):305-310 Wang X Q, Li F, Liu J L, et al. Transcriptomic, proteomic and metabolomic profiling unravel the mechanisms of hepatotoxicity pathway induced by triphenyl phosphate (TPP)[J]. Ecotoxicology and Environmental Safety, 2020, 205:111126 Kang W L, Li X K, Sun A Q, et al. Study of the persistence of the phytotoxicity induced by graphene oxide quantum dots and of the specific molecular mechanisms by integrating omics and regular analyses[J]. Environmental Science&Technology, 2019, 53(7):3791-3801 Xia P, Peng Y, Fang W D, et al. Cross-model comparison of transcriptomic dose-response of short-chain chlorinated paraffins[J]. Environmental Science&Technology, 2021, 55(12):8149-8158 Song Y, Villeneuve D L, Toyota K, et al. Ecdysone receptor agonism leading to lethal molting disruption in arthropods:Review and adverse outcome pathway development[J]. Environmental Science&Technology, 2017, 51(8):4142-4157 Baralic K, Živancevic K, Božic D, et al. Potential genomic biomarkers of obesity and its comorbidities for phthalates and bisphenol A mixture:[QX (Y12#] In silico toxicogenomic approach[J]. BIOCELL, 2022, 46(2):519-533 Yu F F, Zuo J, Fu X L, et al. Role of the hippo signaling pathway in the extracellular matrix degradation of chondrocytes induced by fluoride exposure[J]. Ecotoxicology and Environmental Safety, 2021, 225:112796 Peng T, Wei C H, Yu F B, et al. Predicting nanotoxicity by an integrated machine learning and metabolomics approach[J]. Environmental Pollution, 2020, 267:115434 Yamane J, Aburatani S, Imanishi S, et al. Prediction of developmental chemical toxicity based on gene networks of human embryonic stem cells[J]. Nucleic Acids Research, 2019, 47(3):1600 Neves B, Moreira-Filho J, Silva A, et al. Automated framework for developing predictive machine learning models for data-driven drug discovery[J]. Journal of the Brazilian Chemical Society, 2021:110-122 Chen H M, Engkvist O, Wang Y H, et al. The rise of deep learning in drug discovery[J]. Drug Discovery Today, 2018, 23(6):1241-1250 Seal S, Yang H B, Vollmers L, et al. Comparison of cellular morphological descriptors and molecular fingerprints for the prediction of cytotoxicity-and proliferation-related assays[J]. Chemical Research in Toxicology, 2021, 34(2):422-437 -
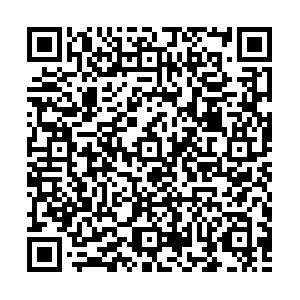
计量
- 文章访问数: 2939
- HTML全文浏览数: 2939
- PDF下载数: 171
- 施引文献: 0